Should we be treating algorithms the same way we treat hazardous chemicals?
Algorithms have the potential to cause injury illness and death. Yet we’ve hardly scratched the surface of how to assess and manage the…
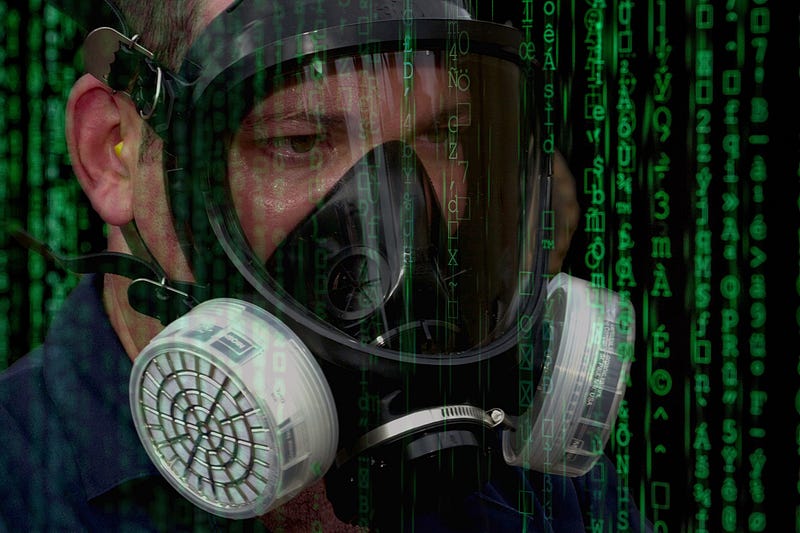
At first blush, algorithms and hazardous chemicals have precious little in common. One is created out of computer code, and exists in cyberspace; the other is made up of atoms and molecules, and forms a very real part of the world we physically inhabit.
Yet despite these differences, both have an uncanny ability to impact our lives in ways that are neither desirable, nor always easy to predict. And because of this, there are surprising similarities in how the risks associated with the development and use of algorithms might be assessed.
The responsible development and use of algorithms has, of course, been a focus of discussion for some time. At one extreme there’s the well-publicized yet rather speculative risk of superintelligent machines wiping out life on earth. On the other, there are the closer-to-home risks of algorithms deciding who lives and who dies in a self-driving car crash, or the dangers of them reflecting the racial and gender biases of their creators.
These and other possible “algorithmic risks” are prompting a growing number of researchers to ask what might go wrong as we grapple with the fickle line between desired benefits and unacceptable harm.
And yet the field of algorithmic risk still has a long way to go before research can reliably be used to make informed risk decisions. And this is where the parallels between algorithms and chemical risk assessment have the potential to accelerate progress.
The risks of algorithmic “predictive inequity”
Reading a recent headline in in MIT Technology Review, I was struck afresh by how similar the challenges in algorithmic risk research are to many of those the chemicals community have struggled with for decades.
Reporting on new research on self-driving cars and “predictive inequity”, the headline proclaimed “Self-driving cars may be more likely to hit you if you have dark skin.” This is a startling, unsettling claim. It’s also deeply worrying if you live somewhere where self-driving vehicles are commonplace on public roads, and you have dark skin.
Social media coverage was even more decisive in how the paper was interpreted. Kate Crawford, co-founder of the AI Now Institute tweeted “Guess what? Study shows that self-driving cars are better at detecting pedestrians with lighter skin tones. Translation: Pedestrian deaths by self-driving cars are already here — but they’re not evenly distributed.”
The paper itself is a little more guarded in its conclusions, as it couches indications of potential bias in qualifying caveats. Yet it does raise the possibility that the pedestrian-detection systems potentially used in self-driving cars may be better at identifying and avoiding people with lighter colored skin, compared to those with darker skin tones.
This is what the authors refer to as “predictive inequity” or the possibility that technologies using such systems could be biased toward harming people based on the color of their skin.
Given the seriousness of these findings, it’s not surprising that people are worried that self-driving cars may have baked-in biases. Yet as researchers in other areas of risk have discovered, knee-jerk reactions to seemingly-startling results rarely result in socially beneficial outcomes.
So how should developers and others be conducting, weighing, and making sense of research into the potential risks of algorithms?
Part of the answer lies in how risks in other domains are assessed and managed — and in particular, what can be learned from how we assess and manage the potential risks from commercial chemicals.
Navigating chemical risks
Commercial chemicals — those chemicals that are intentionally manufactured and used to make the things our lives so-often rely on — are often a double-edged sword when it comes to risks and benefits. Yet despite the aspirations of some to lead a “chemical-free” life, manufactured substances are the backbone of modern society.
Commercially produced chemicals are used to produce the clothes we wear, the houses we live in (and what we put in them), the cars we drive, the roads we drive on, the electronic devices we can’t live without, the food we eat, the services we rely on, and much more besides.
Without commercial chemicals, it’s not too much of a stretch to say that modern society as we know it would collapse.
And yet, this dependency comes at a price. If not used responsibly, commercial chemicals and their products can disrupt the climate, destroy ecosystems, clutter oceans, cause disease and death, and an awful lot more.
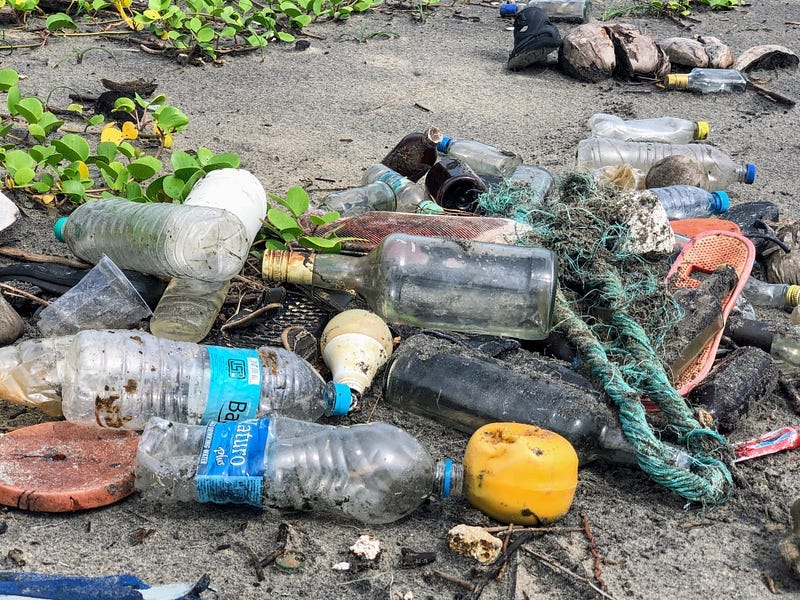
Because of this tension between utility and impact, businesses and regulators have had to learn the hard way how to understand and manage the risks of these substances, so that users can take advantage of their very-real benefits.
Of course, it should be pointed out that, despite substantial progress over the past century, they still have a lot to learn.
For instance, scientists and regulators still struggle to understand and manage the potential effects of chronic exposure to some chemicals — endocrine disruptors for instance. And there remain many ways that chemicals interact with our bodies and the environment that researchers don’t yet fully understand — including the effects of exposures within critical periods of development, such as in the first trimester of pregnancy.
Yet despite these challenges, scientists have developed an impressive array of methods and tools for assessing and managing risks from chemical exposures. And many of these have an unexpected relevance to the potential risks of algorithms.
The curse of inadequate evidence
At the hear of risk assessment is evidence, and how it’s used; irrespective of whether the risk is from chemical exposure, algorithms, or some other source. And one of the biggest dangers in making sense of risk is ignoring, misinterpreting, or misusing evidence.
This danger becomes especially relevant where limited or poor quality evidence is combined with assumptions about possible risks. This is where cognitive biases and mental shortcuts all too easily easily fill in the data gaps and create a compelling but often-deceptive perception of reality.
When risk studies come along that seem to support intuitive fears or deeply held beliefs it’s all too easy to take them at face value. And it’s tempting to use them to drive decisions and regulations that feel like they’re the right thing to do.
Yet this reliance on assumptions and beliefs inevitably leads to decisions that are based less on evidence, and more on how we perceive the world to be.
These are natural tendencies, and we’re all subject to them — especially when the health or life of someone we care for is at stake. Yet they can all-too-easily lead to “cherry picking” studies and data that seem to confirm prior assumptions, irrespective of their validity.
Because of this, communities working on health risks have developed intricate ways to avoid human bias from interfering with how research is conducted and interpreted.
Despite this though, it’s all-too-common for well-meaning groups (including researchers) to fall into the trap of unquestioningly accepting research that supports their prior beliefs, while rejecting studies that don’t.
This is seen for instance in communities that refuse to have their children vaccinated because they “cherry pick” misleading and even discredited research to support their position. It’s also seen in the ways that questionable studies are spun to elicit fear around the use of genetic modification in food.
It’s cases like these that lead to risk professionals being deeply skeptical about using single studies, and relying on prior assumptions to drive decisions that potentially affect people’s lives. And one way this skepticism is demonstrated is through the many protocols and initiatives in place around the world to avoid bias creeping into risk assessments.
Many of these protocols come across as onerous and pedantic to the uninitiated. And to be fair, there are plenty of researchers who feel the same way. Yet they save lives. And they do so by helping to avoid well-meaning but potentially disastrous decisions being made on the flimsiest of evidence.
businesses and regulators have had to learn the hard way how to understand and manage the risks of commercial chemicals so that users can take advantage of their very-real benefits
One of the better-known initiatives that bring checks and balances to the process of health-related risk assessment is the Cochrane network.
The Cochrane initiative is, in the words of the network, committed to “improved health where decisions about health and health care are informed by high-quality, relevant and up-to-date synthesized research evidence.”
Here, the Cochrane Handbook for Systematic Reviews of Interventions is something of a gold standard when it comes to evaluating research into how substances potentially affect people’s health. It’s long, it’s meticulous, it’s demanding. And it works.
Yet it doesn’t address research into the health and safety implications of algorithms and how they’re used.
This is of course no surprise — the handbook is, after all, designed to address more conventional health risks. Yet if algorithms such as the ones in the predictive inequity study above have the potential to lead to harm if used inappropriately, there’s an argument to be made for them being subject to similar levels of rigor.
This is especially true if there’s a risk of over-enthusiastic interpretation of research on algorithmic risks leading to decisions that appear naïve, misguided, and ultimately harmful, with hindsight
Thinking of algorithms as chemicals
Given this, it’s worth asking whether the methods and frameworks behind chemicals risk assessment be applied to algorithms. In other words, is there a need for an equally rigorous set of checks and balances for algorithmic risk research?
Of course, an algorithm is not a chemical. It doesn’t have a physical form, nor a chemical identity that determines how it interacts with biological systems. Yet like many chemicals, algorithms have the potential to endanger lives if not developed and used responsibly.
And once you get beyond the different mechanisms behind how they act, the analogy between algorithms and chemicals becomes intriguingly compelling.
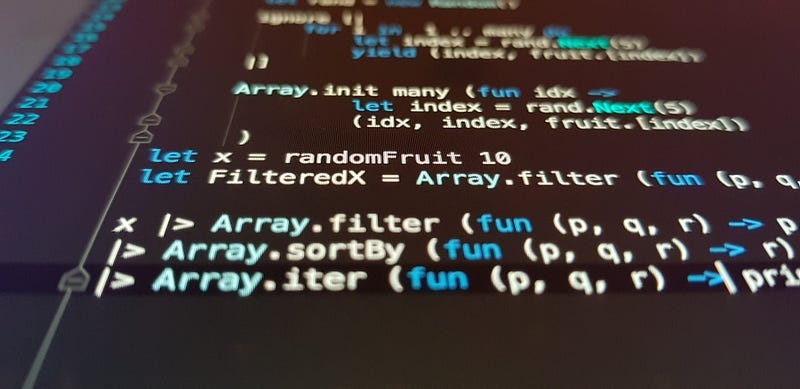
This is where there are a number of concepts embedded within chemical risk assessment that are potentially useful.
Five of these stand out as being especially helpful. These include:
Understanding the difference between the potential for something to cause harm versus the probability of harm occurring;
Identifying events that translate potential harm to actual harm;
Making sense of the consequences of a given risk;
Evaluating how much “stuff” is needed to cause a given level and type of harm; and
Using checks and balances to ensure rigor and lack of bias in how evidence is used to inform decisions.
These concepts are deeply embedded in how researchers, manufacturers and regulators assess risks from commercial chemicals. And they are directly applicable to research on algorithm-based risks.
Differentiating between hazard and risk
If something is hazardous — that is, if it has the potential to cause harm — why wouldn’t risk assessors recommend that it isn’t used? Presumably it’s better to be safe than sorry when it comes to working with potentially dangerous things, whether they are chemicals or algorithms?
Unfortunately risk professionals learned centuries ago that conflating hazard with risk ultimately doesn’t help anyone, as everything has the potential to cause harm buried somewhere within it. And as a result, one of the pillars of modern risk assessment is navigating the difference between hazard and risk.
Of course, many chemicals in our lives are relatively benign. But some have a high potential to cause harm — they’re highly hazardous. The gasoline in your car for instance, or the bleach under your sink.
Yet unless this potential is somehow unleashed, the risks associated with these substances remain low.
It’s a little like the difference between observing an enraged grizzly bear from a distance (high hazard but low risk), and coming face to face with the same bear on the ground (definitely high risk).
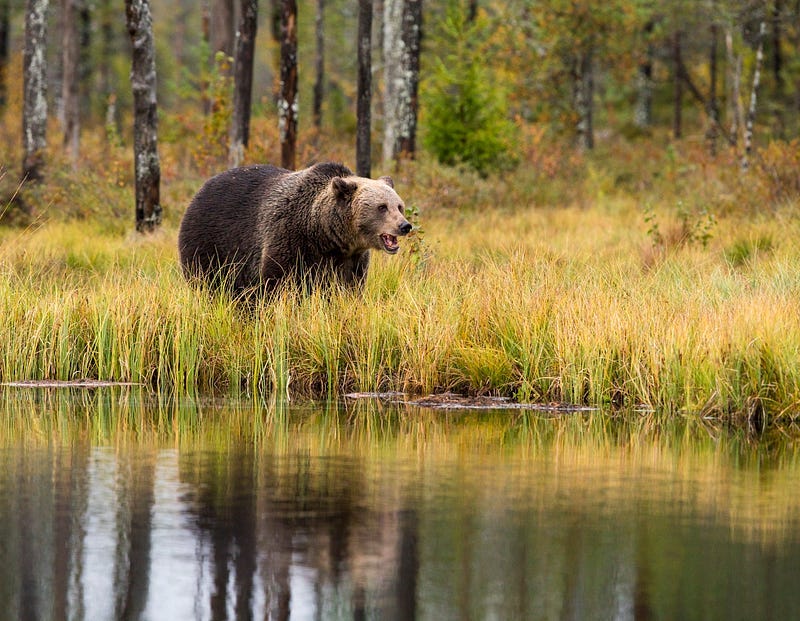
In the case of bleach, the risk is negligible until someone does something stupid, like drink it. And the gasoline in your car only becomes a risk if it is spilled, the car malfunctions, or there’s a crash.
This same distinction between the potential to cause harm and actual risk can be applied to algorithms.
For instance, research on a hypothetical use of an algorithm can indicate that, if circumstances are right, it could cause serious harm. If a particular algorithm could conceivably be used to control aircraft or medical devices for example, and it’s shown to have serious flaws, it clearly represents a hazard.
Yet this hazard will only become a risk if the algorithm is deployed without appropriate modifications and safeguards.
This distinction between hazard and risk is highly relevant to algorithmic risk. But to make sense, it needs to be accompanied by an understanding of what leads to hazard being translated into risk in the first place.
Algorithmic exposure
In the chemical world, it’s exposure that makes the difference between hazard and risk. No exposure means no risk, even if a chemical is potentially deadly.
But what’s the equivalent to “exposure” when it comes to algorithms? Is there even an equivalent?
I think there is. Users of systems and devices are effectively exposed to algorithms if they are affected by the decisions those algorithms lead to. And it’s not just users. Anyone who is potentially impacted by the deployment of an algorithm can be thought of as being exposed to it in some way.
For example, and again going back to the predictive inequity paper, a poorly-designed obstacle seek-and-avoid system that’s used in self-driving cars “exposes” pedestrians to a potential hazard. In this case, the exposure doesn’t seem to be uniform, but is instead potentially weighted toward people with darker skins.
This is where the concept of “algorithmic exposure” becomes valuable as a way of assessing whether a potential to cause harm under very specific conditions — in the lab or under computer simulations for instance —is likely to be converted into an actual risk in the real world. In some cased it will, but not always.
For instance, in the predictive inequity paper, the object-identification algorithms being studied were representative of real-world applications. But they were not identical to the full-blown systems currently being used in self-driving cars.
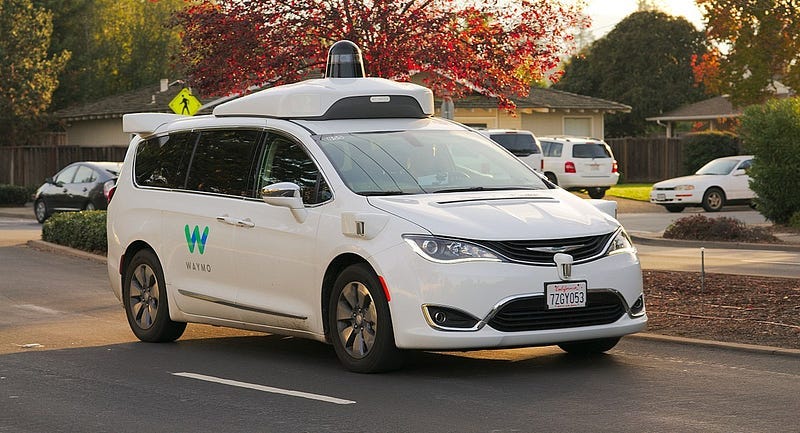
These on-the-road systems complement optical imaging with LIDAR detection and proprietary machine learning. And they’re designed to detect whole objects based on their size, shape, and differentiation from what’s around them.
Because of this, it’s unlikely that the systems used in on-the-road self-driving cars would exhibit precisely the same behaviors as the researchers saw. At best, it’s unclear whether the algorithmic hazards observed would lead to algorithmic risk, because there is no clear algorithmic exposure route.
This in no way negates the research, which established an important potential for poorly conceived systems to cause harm. But it does underline the need to identify viable exposure pathways in extrapolating research findings to real-life scenarios.
But where such pathways do exist, how do we make sense of how much harm might occur, and — importantly — what sort of harm might result?
This is another question that is deeply embedded within chemical risk assessment. Evidence of risk is is important. But it’s just one step in the process of evaluating the possible consequences of exposure.
Parsing the potential consequences of algorithmic hazards
The potential consequences of being exposed to harmful chemicals can vary from the mundane — low-level, reversible effects for instance, that are more an irritation than a serious threat — to the severe, including disease, disability, and even death.
This is where consequences matter, even if the probability of harm occurring is high.
Most people for instance wouldn’t consider intestinal discomfort to be on the same level as bowel cancer, even if the chances of each occurring after an exposure was similar. Likewise, there’s a world of difference between indigestion and heart failure.
Consequences matter in chemical risk assessment. And there’s no reason why they shouldn’t matter in the same way when making sense of algorithmic risks.
The question is, how do we begin to make sense of, and weigh the relative importance of, different types of risks that may result from how algorithms are designed and used?
If we’re serious about ensuring the safe and beneficial development and use of algorithms, we need to treat them with the same rigor and respect that we do the chemicals that our lives depend on
Just as with chemical exposures, algorithms are likely to be responsible for many types of possible harm. This is especially true when algorithms are used to support decisions and behaviors that are directly tied to health.
But this is also true where algorithms lead to people being threatened in other ways. For instance, how do we begin to parse the consequences of algorithms that result in people’s livelihood being threatened? Or their liberty, dignity, and self-respect?
Of course, the specific ways in which harm is caused will be quite different between chemicals and algorithms. And yet, the underlying idea that the type of harm is as important as the probability of harm occurring, is common to both.
In chemical risk assessment though, this is not the end of the process. Once there is an understanding of what harm might be caused and how, there’s a final, crucial, step before the risk can be assessed.
This involves modeling the relationship between exposure and response (or, more accurately, dose and response), so that the consequences of different exposures can be predicted. And it’s this step that’s at the heart of informing what action is needed to address a particular risk.
Algorithmic exposure-response
Dose response models allow chemical risk assessors to estimate how much “stuff” is likely to lead to what sort of impact, within a large population. In many ways it’s a crude science as the data these models are based on are usually messy — but it’s getting better.
Yet even with the messiness, dose-response modes are essential for assessing chemical risks and managing them.
And this raises the question of whether similar predictive models are possible to construct for algorithms.
Using the predictive inequity paper as an example, it’s possible to think of “exposure” as the likelihood of pedestrians encountering self-driving cars using object-detection algorithms that are biased against people with darker skin tones. And in the same vein, it’s possible to equate “harm” to such encounters leading to injury or death.
From here, it should be possible in principle to predict the chances of harm occurring for a given level of encounters with cars running the suspect algorithm.
This would be the algorithmic equivalent of a chemical dose-response relationship — an “algorithmic exposure-response relationship” if you like. And its power lies in the ability to estimate the probability of pedestrians with darker skins preferentially being harmed by self-driving cars.
Such an estimate — even if it’s possible — isn’t sufficient to determine whether a given level of risk is acceptable or not. It merely provides a number that developers, regulators, advocacy groups and others can use in making informed decisions as they grapple with what an “acceptable” level of risk versus an “unacceptable” level is.
Of course, the ideal is that there’s no such thing as acceptable risk. Yet when it comes to chemical risks, we’ve had to come to terms as a society with the reality there’s no such thing as zero risk. And this means that, if we want to enjoy the benefits of the “stuff” that commercial chemicals enable, we also have to be clear-eyed about how much risk we as a society are willing to put up with.
In effect, there’s always a risk of not developing or using a product, that needs to be balanced against the risks that using it might entail. And this is where being able to predict risk is especially helpful.
In the case of skin-tone bias, there’s a very strong case to be made that there is no such thing as “acceptable” risk. And without doubt, developers and manufacturers should be striving to achieve this. At the same time, human drivers have been shown to exhibit a similar bias. And so even here, there’s a tradeoff between the relative risks of deploying good but not perfect technologies, and accepting the current state of affairs.
This example highlights just how challenging risk decisions can become. And it underlines the importance of using solid evidence in making them.
But even if you can estimate probabilities of specific types of harm occurring, how do you know that the evidence you are using, and the procedures you’re relying on, are trustworthy?
Weighing the evidence
There’s an old computer adage that says “garbage in, garbage out.” It’s an adage that deeply applicable to chemical risk assessment, as garbage evidence leads to garbage risk assessments. And by inference — and coming full circle — it’s equally relevant to understanding and acting on the potential risks of algorithms.
This brings us back to the approaches that researchers and others use to make sense of evidence.
In chemical risk assessment, there is often a deep respect for individual studies that push the boundaries of what is known, and a parallel deep skepticism around using these studies in isolation for making decisions.
It’s a skepticism that’s based on the understanding that a single study can be useful, but inevitably is just one small piece of a much larger puzzle. And to treat such a piece as if it’s the whole picture is, at best, misleading.
This skepticism is also based on the reality that most studies have their limitations. And here, risk researchers often rely on a number of rules of thumb to make sense of new research.
These rules of thumb include (but are far from limited to) being on the lookout for implicit bias in studies, treating research that is statistically weak with caution, not trusting conclusions based on a single study, and relying heavily on the emerging weight of evidence from multiple studies.
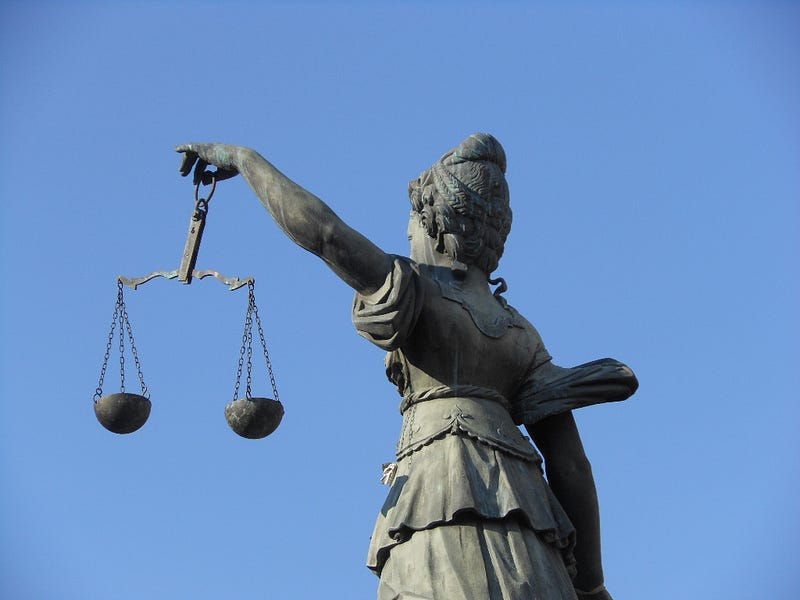
Risk researchers also tend to put a high premium on checks and balances in research, and are suspicious (but not off-the-cuff dismissive) of studies where the researchers may have a vested interest in the outcomes.
In the world of chemicals risk assessment, these approaches to how research is conducted and the use of evidence lead to a level of rigor which, while far from perfect, does help to avoid serious errors.
And there’s no reason why similar approaches couldn’t be extended to research on algorithm-based risks.
In fact, I would go so far as to say that they must be, lest we end up building our understanding of algorithmic risks on an evidentiary stack of cards that collapses at the first hurdle.
Learning from chemicals risks
Of course, at the end of the day, algorithms and chemicals aren’t the same. And chemical risk assessment methods are not nearly as robust as we’d like them to be.
And yet, there’s still a lot to be learned from chemicals about how to evaluate the potential risks of algorithms as we try to develop and use them safely.
As a starting point, we need to establish robust ways of thinking critically about algorithmic risk. And we need to develop rigorous checks and balances that support evidence-based decisions on algorithm safety.
We also need to develop better ways of ensuring algorithms don’t lead to risks that are all too easily overlooked — such as preferentially affecting the lives and livelihoods of people of color, or discriminating on the basis of gender or religion.
Multiple efforts are already underway here. The AI Now Institute for instance is doing sterling work on the safe and responsible development and use of algorithms and artificial intelligence more generally. And organizations like Deloitte are digging into novel approaches to managing algorithmic risk.
There’s also a vibrant and growing community of researchers that are grappling with the potential downsides of algorithms that look good on the surface, but have the potential to lead to discrimination and harm.
But these are only scratching the surface of what needs to be done if algorithms — and machine learning and AI more broadly — are to be developed and used as safely and as beneficially as possible.
This is where there’s more that can be learned from how we assess and manage chemical risks than we might imagine.
If we’re serious about ensuring the safe and beneficial development and use of algorithms, we need to learn how treat them with the same rigor and respect that we do the chemicals that our lives depend on.
Unless, that is, we’re content to repeat the mistakes of the past as we reinvent the future.