Flattening the learning distribution curve using ChatGPT
Could innovative uses of generative AI transform how we teach to the edges of the learning distribution curve?
I’ve given a few talks recently where I mention the idea of generative AI “flattening the learning distribution curve”. It’s a concept that feels intuitively right. But I must confess that I sometimes come away from these events thinking to myself “is this really a thing?”
It turns out that the concept does make sense — and it’s not even a particularly new one in educational thinking writ large. But to understand it in the context of the generative AI takes a bit of unpacking.
Many formal education settings use a “one-to-many” approach to teaching, where the educator is the “one” and the students are the “many”. Yet in this approach educators have few options but to teach to the mean of student potential and responsiveness.
When we teach to the mean, we’re attempting to increase overall student success to the best of our ability. But even the most talented teacher is limited in what they can achieve with large classes and limited additional support. I’m thinking in particular of large undergraduate classes here, where it’s not uncommon to be teaching hundreds of students at the same time. But the approach also applies to teaching from K - 12.
As we teach to the mean as educators, we can’t help but struggle to serve students toward the edges of the learning distribution curve. These include students who find it harder than others to learn new material within a specific context, as well as those who find the teaching environment too slow and limited to meet their needs.
The result is a student success distribution curve something like the one below, where students with learning potential and responsiveness to teaching style at the edges of the distribution suffer.
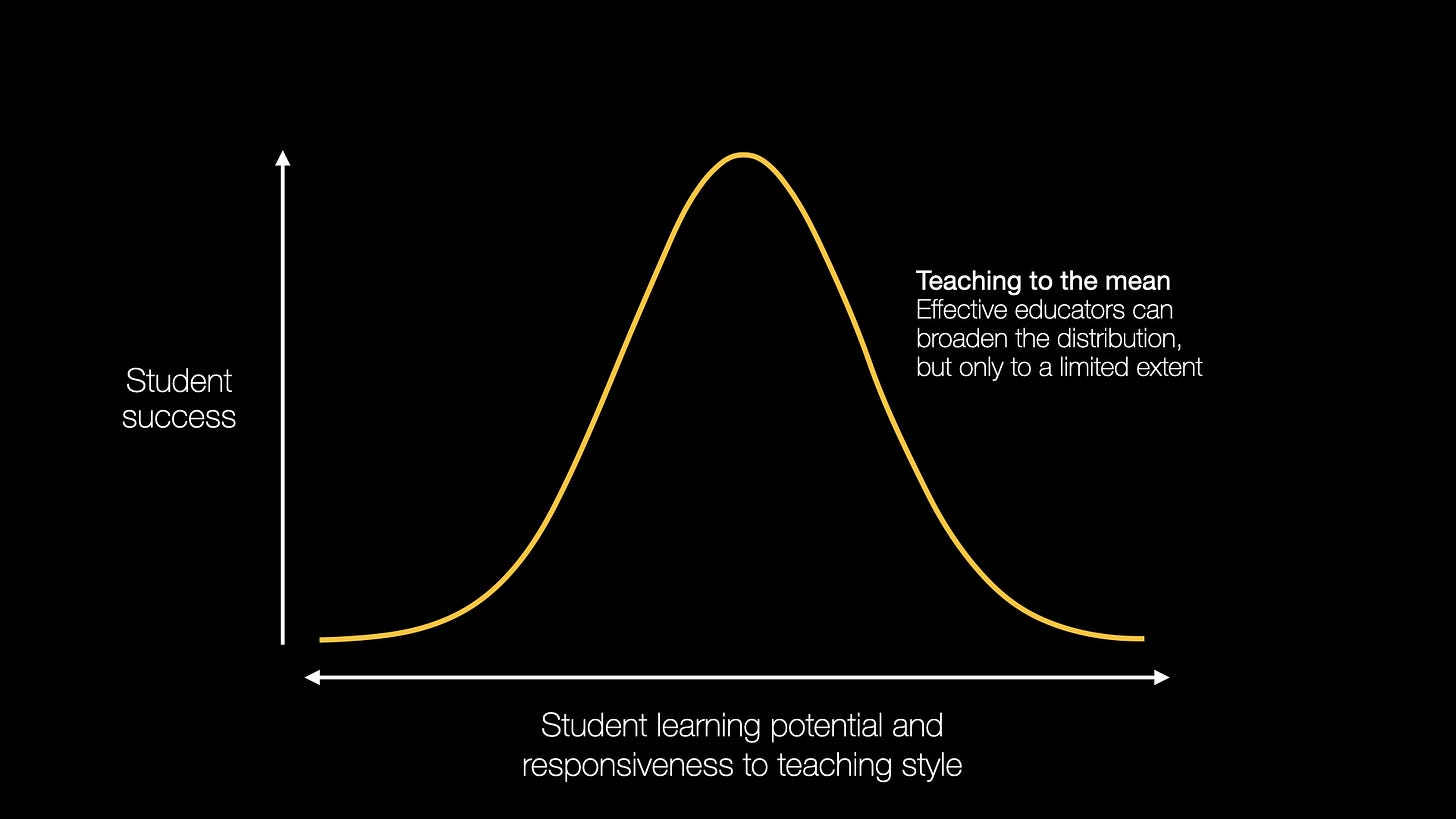
This is, admittedly, a somewhat stylized and over-simplified illustration. But it does capture the idea of a learning distribution curve with a clear mode and distinct edges.
Effective educators can, of course, broaden this distribution. And by using teaching tools and methodologies that differentiate between students of differing abilities, they can stretch it out even further. But in a one-to-many environment, there are always students at the edge of the curve who have the potential to achieve more, but don’t have the opportunity to do so.
Here it’s worth mentioning that one approach to dealing with the edges of the distribution curve is to simply get rid of them — usually by selecting students who are most likely to succeed within a given environment. It’s an approach to education that ensures a great match between teacher and students — and one that maximizes success within well defined bounds. But it does so by excluding students at the edges.
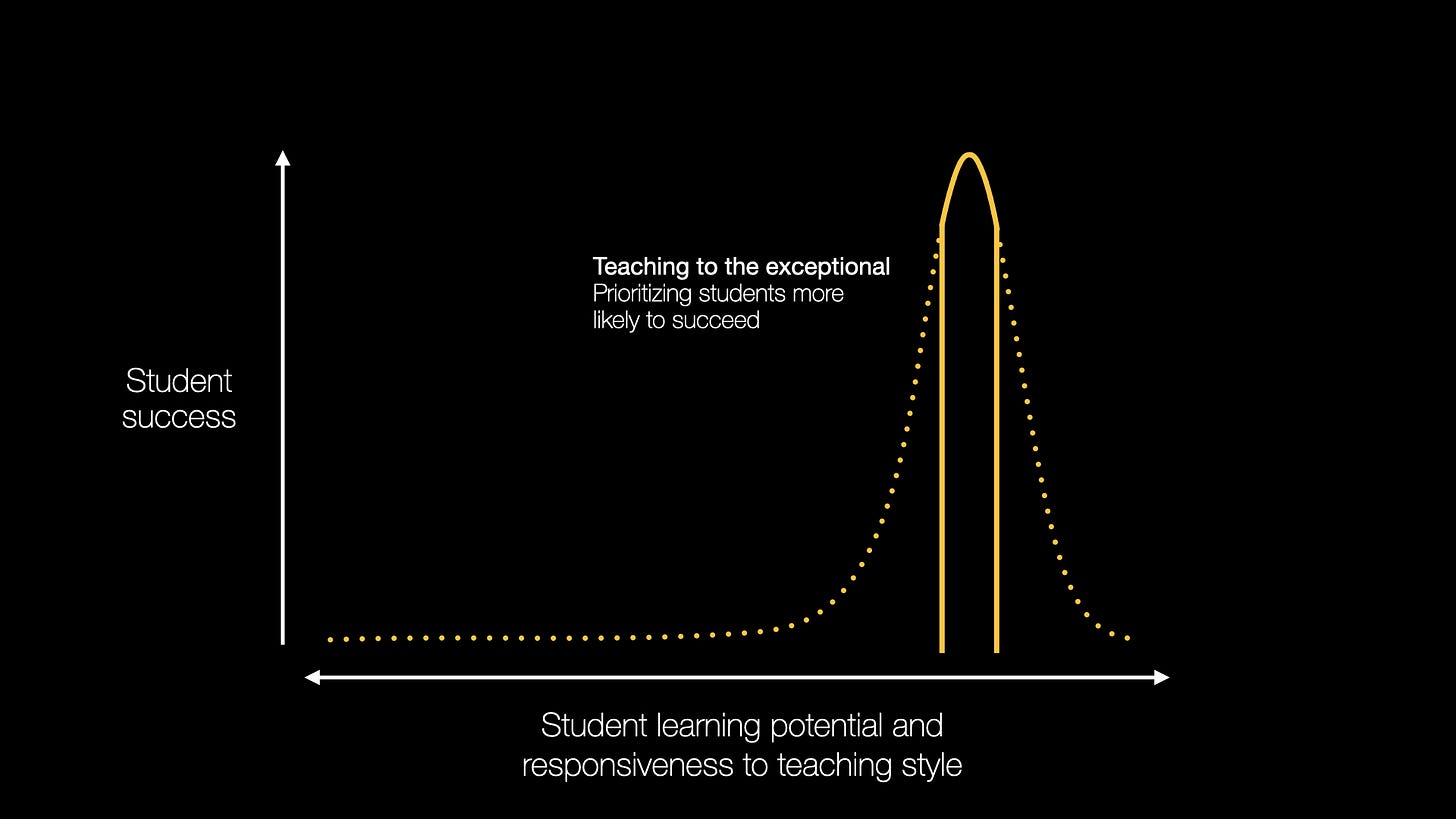
This is not an approach that sits well with me as an educator. It’s also not one that aligns with the ethos of learning and education at a public university — especially one like Arizona State University where I teach, and where we measures success by whom we include, not by whom we exclude.
But if narrowing the distribution isn’t an option, and broadening it only goes so far, what are other ways of ensuring the success of as many students as possible in a one-to-many teaching environment?
This is where the concept of flattening the distribution curve comes in.
If it was possible to elevate student success at the edges of the distribution, the result would be greater student success across all levels of learning potential and responsiveness to teaching style.
And here, AI is looking increasingly promising as an effective distribution curve flattener.
Over the past twelve months there’s been increasing interest in generative AI — ChatGPT and similar text-based AI chatbots in particular — being used to enhance and scale learning. And it’s becoming increasingly clear that these tools have the potential to help flatten the learning distribution curve by augmenting teaching at the edges.
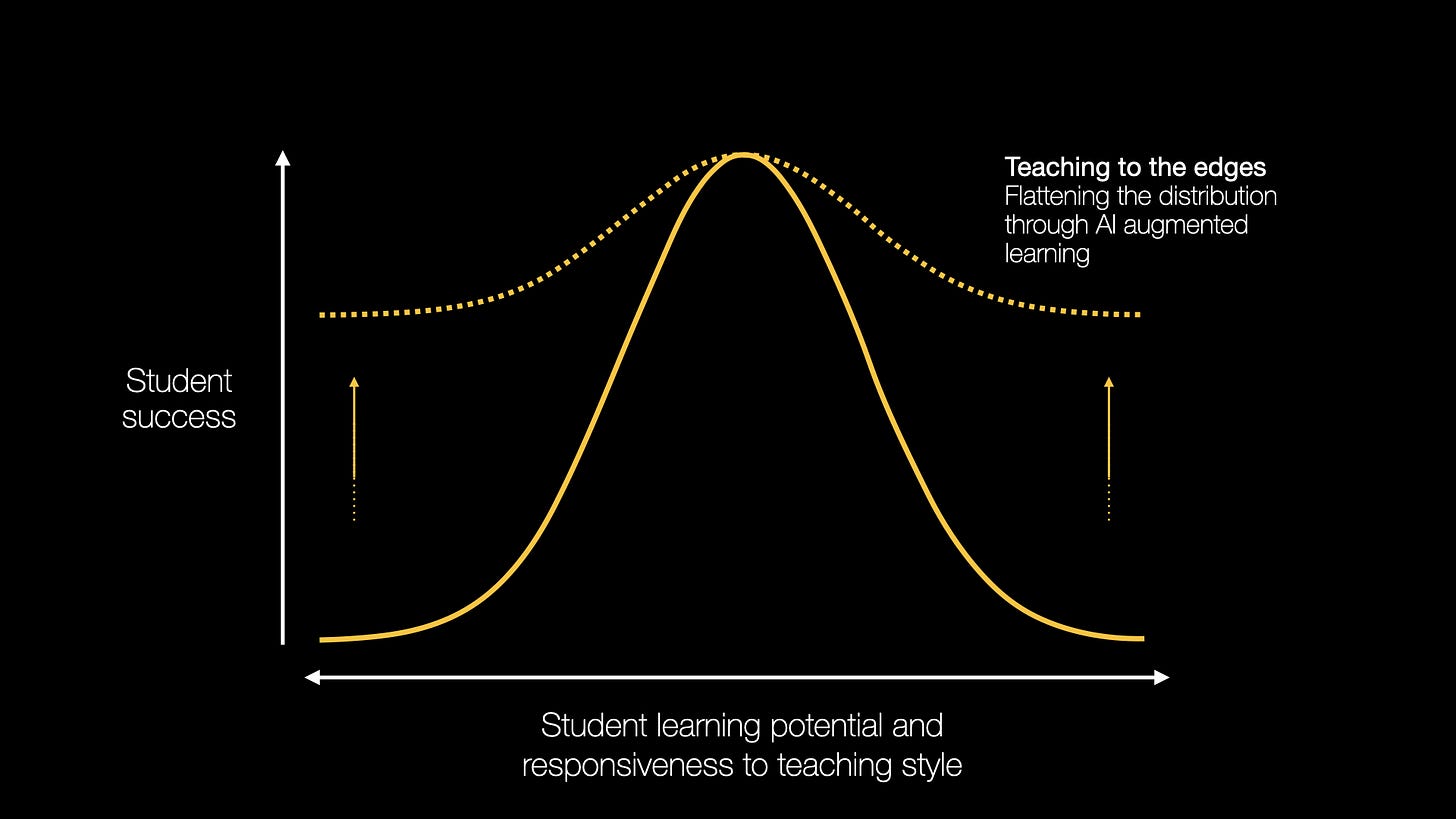
We’re still at the very early stages of understanding all the ways that AI can enhance teaching. But evidence is already emerging of instructors using generative AI to tailor learning to individual students, as well as students themselves using AI in creative ways to augment their own learning.
In my own classes I now have numerous examples of assignments that use ChatGPT to allow students to learn on their own terms and at their own pace — all within the broader context of a specific set of learning objectives. In the course I teach on prompt engineering using ChatGPT for instance, two of the assignments ask students to have a detailed discussion with the chatbot on bias and failure modes. While I set the assignment, each conversation is tailored to an individual student’s interests, questions, level of understanding, and learning preferences. As a result, every student has a highly personalized learning experience.
A similar level of personalized learning occurs in a later assignment where students are asked to engage in a Socratic dialogue with ChatGPT around a particular topic. Of course the absolute accuracy of ChatGPT’s responses cannot be guaranteed. But this adds to the personalized learning environment as each student is challenged to question and test what they are exposed to as part of a dialogue that’s unique to them.
These are just two simple ways in which I’m beginning to see generative AI flatten the learning distribution curve in my own classes. I’m also hearing of cases where students are using ChatGPT as a real-time ideas-translator and explainer in class; where they’re augmenting their course material through discussions with AI chatbots; and even where they’re using generative AI to explain well-meaning but poorly articulated assignments.
I suspect these are just the tip of an AI-augmented distribution-flattening iceberg. With creativity, imagination, and some out of the box thinking, I pretty sure there are ways to massively expand how generative AI is used to flatten the learning distribution curve. And this is with todays limited and flawed AI tools. Who knows what the next generation of breakthroughs will bring?
The only limit here seems to be the imagination of educators and the willingness of educational establishments to experiment with how AI is used to augment teaching, and to elevate student success across the board.
Of course, this also depends on navigating the limitations and challenges posed by AI — including bias, persuasive incorrectness, and a whole host of intellectual property issues.
But even within this complex environment it’s hard to see AI as anything but a game changer as we develop the tools to flatten the learning distribution curve and elevate student success in ways that simply haven’t been possible before.
While I agree with your excitement about the possibilities, it's the teachers I worry about. Will they be given a good lot of time to experiment and learn before being expected to deploy the technology in their class rooms, or will these possibilities merely be added to the load they already carry? As has usually been the case.